Can you confidently predict your business’s sales trajectory in a volatile market? Inaccurate sales forecasts can lead to overstocking, understocking, misallocated resources, and missed revenue opportunities.
Traditional methods, often reliant on gut instinct and static historical data, need help to adapt to the dynamic factors influencing today's markets. AI-driven sales forecasting provides a data-centric solution that leverages advanced algorithms, real-time analytics, and machine learning models to deliver precise, actionable predictions.
In this article, we’ll have a detailed look into AI forecasting for sales, compare it to traditional methods, explore real-world applications, and provide a step-by-step guide to help you implement AI strategies effectively. Whether you’re just starting or looking to improve your sales operations, this guide will offer actionable insights.
What is AI Forecasting in Sales?
AI forecasting in sales involves leveraging artificial intelligence and machine learning algorithms to calculate and analyze extensive datasets. This enables businesses to predict future sales trends and outcomes accurately. Unlike traditional forecasting methods, which rely heavily on historical data and manual interpretation, AI leverages dynamic data inputs such as real-time consumer behavior, external market indicators, and unstructured data sources like customer reviews or social media trends.
Critical Components of AI Forecasting:
- Machine Learning Algorithms: These algorithms identify complex sales data patterns beyond human recognition. For instance, clustering algorithms can segment customers based on purchase behavior to predict individual sales potential.
- Big Data Analytics: AI can process terabytes of data from diverse sources—CRM systems, website analytics, and market trends—transforming them into actionable insights.
- Predictive Modeling: AI creates predictive models to evaluate "what if" scenarios, such as the impact of a price increase or a marketing campaign on sales.
Real-World Application:
A global e-commerce platform like Amazon might use AI forecasting to anticipate demand surges during festive seasons. For example, by analyzing previous sales data, competitor pricing, and weather patterns, the platform can accurately predict the demand for specific products and adjust inventory levels accordingly.
Why is AI-Driven Sales Forecasting Better Than Traditional Methods?
AI-driven sales forecasting outperforms traditional methods across several dimensions. McKinsey has found that businesses using AI-powered forecasting have reduced forecasting errors by 10-15%.

For example, a retailer relying on traditional forecasting may miss out on sudden spikes in demand for specific products due to viral trends. AI, on the other hand, can quickly identify and act on these shifts.
A Step-by-Step Guide to AI-Enhanced Sales Forecasting
Integrating AI into sales forecasting involves a systematic approach to ensure maximum accuracy and usability.
1. Define Forecasting Objectives
Start with clear goals. Are you forecasting overall revenue, regional sales, or product-specific demand? Defining the scope ensures the AI model is tailored to your needs.
2. Data Collection and Preparation
Gather data from multiple sources:
- Internal Data: CRM, ERP, historical sales.
- External Data: competitor pricing, market trends, social media insights.
Ensure data cleanliness and consistency—AI models thrive on high-quality, structured data.
3. Select the Right AI Platform
Choose a platform or tool based on your business size and complexity. Enterprise-grade tools like Salesforce Einstein or Microsoft Azure cater to more extensive operations, while smaller businesses might use affordable options like HubSpot’s AI features.
4. Model Training and Testing
Train the AI model using historical data to identify patterns. Validate its predictions against actual sales figures to fine-tune its accuracy. For instance, testing reveals that the model needs adjustments to better account for regional variances.
5. Deploy and Monitor
Integrate the AI model into your sales processes. Regularly monitor its performance and update it with fresh data to keep forecasts reliable.
6. Iterate and Improve
Sales forecasting isn’t static. Continuously refine the model to adapt to new variables, ensuring it stays relevant as markets evolve.
9 Popular AI Sales Forecasting Models or Techniques
AI-powered sales forecasting relies on cutting-edge models to address unique business challenges. Below is an in-depth look at each technique, including how it works, where it excels, and detailed real-life applications.
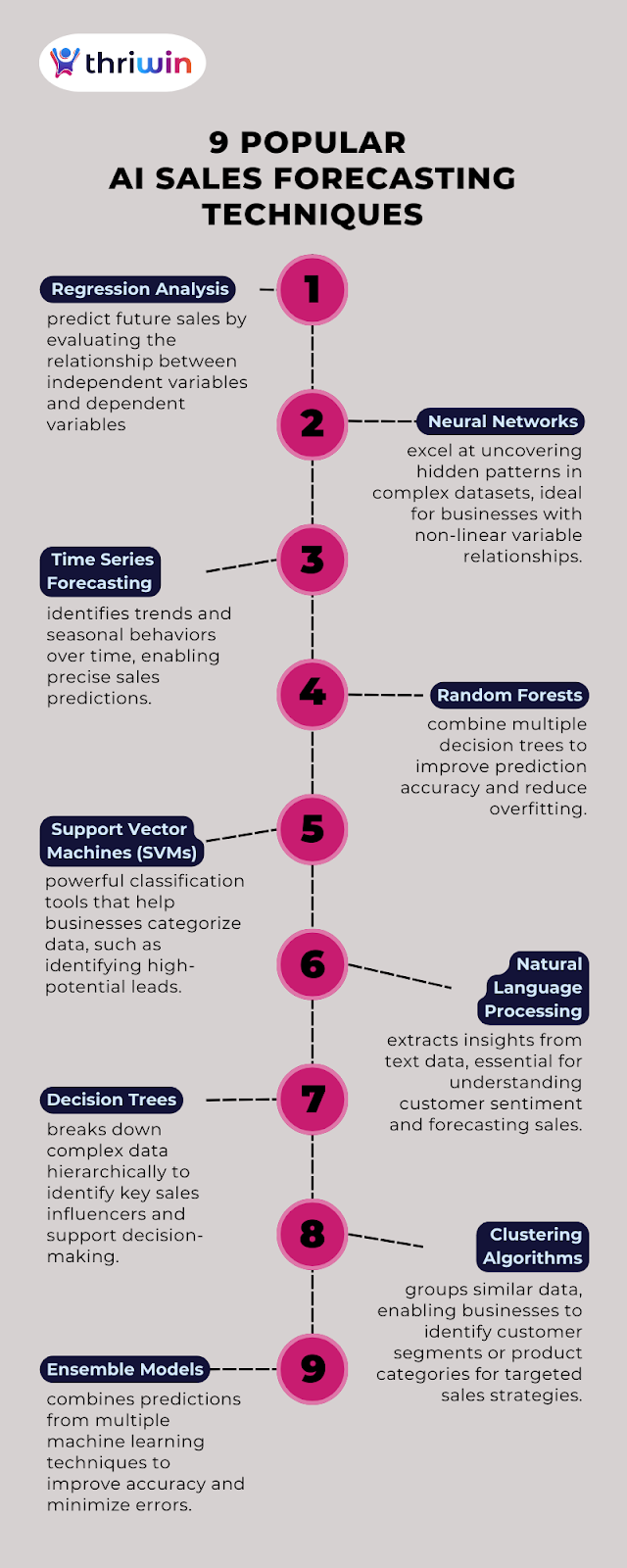
1. Regression Analysis
Regression models predict future sales by evaluating the relationship between independent variables (such as advertising spend or pricing) and dependent variables (such as sales performance).
- How it works: Linear regression examines single-variable relationships, while multiple regression analyzes multiple variables simultaneously to predict outcomes.
- Best Suited for: Businesses looking to understand the relationship between multiple variables and their impact on sales.
- Real-Life Application:
A retail chain wanted to understand how discounts during holiday seasons impacted overall sales. Using regression analysis, they discovered that a 15% discount led to a 30% spike in sales, but only when combined with digital ad campaigns. This insight allowed them to allocate ad budgets more effectively and optimize their promotions. - Limitations: Regression Analysis assumes linear relationships, struggles with complex, non-linear data, and is sensitive to outliers and multi-collinearity.
2. Neural Networks
Neural networks are excellent for identifying hidden patterns in large and complex datasets, making them ideal for businesses with non-linear relationships between variables.
- How it works: The system learns from past data by adjusting the "weights" of connections between neurons to improve accuracy over time.
- Best Suited for: Large-scale enterprises with complex datasets requiring advanced pattern recognition.
- Real-Life Application: A global fashion e-commerce platform used neural networks to predict product demand for eco-friendly clothing lines. By analyzing data such as customer browsing patterns, purchasing history, and demographic information, they identified a growing demand for sustainable clothing. This led to a 25% increase in seasonal revenue.
- Limitations: This technique requires large datasets, significant computational resources, and is prone to overfitting due to its "black box" nature.
3. Time Series Forecasting
Time series forecasting identifies trends and seasonal behaviors, enabling precise sales predictions.
- How it works: Decomposes sales data into trend, seasonal, and noise components to make accurate predictions using methods like ARIMA or Prophet.
- Best Suited for: Industries with seasonal trends or recurring patterns, such as retail, travel, and hospitality.
- Real-Life Application:
A regional airline used time series forecasting to predict ticket sales during festive periods. By analyzing five years of sales data, weather patterns, and holiday schedules, they anticipated a 40% increase in demand for specific routes. The airline adjusted ticket pricing dynamically and expanded capacity on those routes, boosting profitability by 15%. - Limitations: This technique is limited in handling sudden, non-seasonal changes and relies heavily on historical data, which may not always predict future trends.
4. Random Forests
Random forests combine multiple decision trees to improve prediction accuracy and reduce overfitting.
- How it works: Each tree in the forest makes a prediction, and the majority vote determines the final forecast.
- Best Suited for: Businesses needing reliable predictions where multiple variables influence outcomes.
- Real-Life Application:
A consumer packaged goods (CPG) manufacturer used random forests to forecast demand for a new snack product. By analyzing past sales data, competitor pricing, customer demographics, and even weather conditions, they identified optimal stocking levels for different regions. This led to a 20% reduction in overstock costs and increased shelf availability during peak demand. - Limitations: Random Forests are computationally intensive and can overfit when dealing with highly complex datasets.
5. Support Vector Machines (SVMs)
SVMs are powerful classification tools that help businesses categorize data, such as identifying high-potential leads.
- How it works: SVMs separate data into classes using a hyperplane that maximizes the margin between categories.
- Best Suited for: Businesses focused on lead qualification and customer segmentation.
- Real-Life Application:
A B2B technology company used SVMs to classify sales leads as “likely to convert” or “unlikely to convert.” By analyzing factors like email interactions, they optimized their sales funnel through demos attended and CRM engagement; they optimized their sales funnel. As a result, highly probable leads, increasing conversion rates by 18%. - Limitations: Support Vector Machines (SVMs) are inefficient with large datasets and often difficult to interpret in non-linear scenarios.
6. Natural Language Processing (NLP)
NLP extracts valuable information from text data, making it indispensable for understanding customer sentiment and predicting sales outcomes.
- How it works: Models analyze unstructured text data, classify sentiment, and identify emerging trends or recurring themes.
- Best Suited for: Businesses needing insights from unstructured data like customer feedback or social media.
- Real-Life Application:
A global cosmetics brand used NLP to analyze product reviews and social media hashtags. They identified a growing demand for vegan-friendly cosmetics in specific markets. Using this insight, the company adjusted its product lineup and marketing strategy, achieving a 30% sales increase within six months. - Limitations: Natural Language Processing (NLP) requires extensive preprocessing of unstructured text data and may misinterpret context in ambiguous language.
7. Decision Trees
Decision trees use a hierarchical structure to break down complex data into manageable decisions, providing insights into sales influencers.
- How it works: Decision points (nodes) assess scenarios with branching outcomes.
- Best Suited for: Companies seeking a visual, easy-to-interpret approach to forecasting.
- Real-Life Application:
A regional furniture retailer used decision trees to understand how delivery speed, product price, and customer reviews affected sales. By identifying that delivery times under five days significantly boosted conversions, the company prioritized inventory at warehouses near high-demand regions, improving sales by 12%. - Limitations: Decision Trees are prone to overfitting with noisy data, and their performance can vary significantly depending on tree depth.
8. Clustering Algorithms
Clustering algorithms group similar data points, helping businesses identify customer segments or product categories for more targeted sales strategies.
- How it works: Techniques like K-Means clustering group data points based on shared attributes such as spending habits or purchase history.
- Best Suited for: Businesses aiming to segment customers or products for targeted forecasting.
- Real-Life Application:
A subscription-based meal kit company used clustering algorithms to segment customers by dietary preferences. By predicting demand for keto-friendly and vegan meal plans in different cities, the company optimized inventory and reduced food waste by 25%. - Limitations: These depend heavily on the number of clusters chosen and struggle with overlapping data points or ambiguous group boundaries.
9. Ensemble Models
Ensemble models combine predictions from multiple machine learning techniques to improve accuracy and minimize errors.
- How it works: Methods like bagging and boosting aggregate predictions from several models, leveraging their strengths while mitigating weaknesses.
- Best Suited for: Scenarios requiring a combination of forecasting approaches for higher accuracy.
- Real-Life Application:
A logistics company used ensemble models to predict shipping volumes during the holiday season. By integrating time series forecasting for demand trends and random forests for weather impact analysis, they improved delivery route optimization, reducing delays by 15% and cutting operational costs by 10%. - Limitations: Ensemble Models are computationally intensive, and combining too many models can lead to diminishing returns or overfitting.
Choosing the Right Model for your Startup Business
Each AI model has specific strengths, making it essential to align the technique with business needs. For example:
- Use time series forecasting for industries with predictable seasonal patterns.
- Employ neural networks for large-scale, complex data scenarios.
- Leverage NLP to understand and act on customer sentiment.
Selecting the right model—or combining models—can significantly reduce the accuracy and relevance of your sales forecasting efforts.
6 Benefits of AI in Sales Forecasting
- Improved Precision: A study from Gartner in 2023 revealed that firms using AI-based predictive analytics reduced forecasting errors by 50%, translating into more reliable supply routes and decreased wastage.
- Real-Time Decision-Making: Provides instantaneous updates, empowering businesses to respond quickly to changes.
- Optimized Inventory Management: Prevents overstocking and stockouts by accurately predicting demand.
- Personalized Sales Strategies: Identifies patterns that guide targeted marketing and promotions.
- Cost Efficiency: Saves time and labor costs by automating repetitive tasks.
- Scenario Analysis: Simulates various "what if" scenarios to prepare for multiple market outcomes.
Challenges in AI Sales Forecasting
- Data Quality Issues: Poor or incomplete data can reduce accuracy. Businesses must invest in data-cleaning processes.
- Implementation Costs: High upfront costs for tools and training may deter small businesses.
- System Integration: Aligning AI with existing sales workflows can be complex.
- Skill Gaps: Companies often need more technical expertise to manage AI tools effectively.
- Privacy Concerns: Handling sensitive customer data comes with compliance risks.
- Over-Reliance on AI: Blind trust in AI without human oversight can result in strategic missteps.
- Limitation in Application: While AI excels in processing large datasets, it struggles when data is incomplete, sparse, or noisy. In such cases, traditional statistical models like linear regression or moving averages might still outperform AI in terms of accuracy and simplicity. For example, businesses in niche markets with limited historical data may find that AI forecasting provides unreliable results.
Final Thought
AI-driven sales forecasting is a valuable tool that can provide businesses with actionable insights to navigate today’s competitive and rapidly evolving markets. However, its success depends on clean data, skilled implementation, and ongoing human oversight. By addressing the limitations of traditional methods, AI offers unparalleled accuracy, adaptability, and efficiency.
However, it’s essential to approach AI adoption with a clear strategy, ensure data quality, select the right tools, and maintain human oversight. While challenges exist, they are manageable with suitable investments in technology and skills. For decision-makers, the message is clear: embracing AI in sales forecasting is no longer optional—it’s the key to sustainable growth.
Transform Your Sales Forecasting with AI – Partner with Thriwin Today
The future of sales forecasting is here, and it’s AI-driven. Start by evaluating your current forecasting methods and identifying areas where AI can make a difference. Whether it’s optimizing inventory, predicting customer demand, or identifying new market opportunities, AI can help. If you’re ready to transform your sales operations, partner with Thriwin for tailored AI solutions that match your business needs.
Frequently asked questions
- How can AI be used for sales and business forecasting?
It can quickly identify complex patterns, correlations, and trends within the data. For example, AI can analyze historical sales data alongside external factors like market trends and economic indicators to forecast SaaS product demand accurately.
- Which AI model is best for forecasting?
Neural Networks and Deep Learning for Forecasting
Neural networks and deep learning have gained prominence in AI forecasting. They allow the processing of vast amounts of complex data and the identification of intricate patterns. They excel in tasks where traditional methods fall short.
- How to use AI in financial forecasting?
- Consolidate data from different sources.
- Analyze trends and incorporate insights into forecasts.
- Validate assumptions and make accurate data-driven decisions.
- Explore more than one potential scenario, which compromises the quality of insights.
- What is the difference between AI sales forecasting and traditional sales forecasting?
AI sales forecasting is data-driven and dynamic, offering more precise and adaptable predictions, whereas traditional methods are more static and rely heavily on manual input and simpler analytics.
AI Sales Forecasting:
- Uses machine learning algorithms to analyze large datasets, uncover patterns, and make predictions.
- Can adapt dynamically to changes in data.
- Considers complex variables like customer behavior, seasonality, and market trends.
Traditional Sales Forecasting:
- Relies on historical data and human judgment.
- Typically uses basic statistical methods like linear regression or trend analysis.
- Less flexible in adapting to new or sudden changes in the market.
- How can small businesses get started with AI sales forecasting?
- Understand Business Needs: Identify specific goals, such as improving accuracy or identifying trends.
- Choose the Right Tools: Start with user-friendly, affordable AI tools like HubSpot, Zoho, or Salesforce with AI features.
- Gather Quality Data: Collect and organize historical sales data for the AI system to analyze.
- Start Small: Begin with simple AI forecasting models to test and validate results.
- Leverage Expertise: Consult with AI vendors or hire data specialists for implementation.
- Iterate and Improve: Continuously monitor and adjust based on outcomes and new data inputs.
Small businesses can start by using existing platforms that offer AI capabilities rather than building custom solutions from scratch.